It’s good practice to normalize the different variables
Code
d=iris[,-5] # numerical iris data (without speciee) ds=scale(d) # scaled iris data (column-wise)br=seq(-5,7,by=0.5) # set common break points for the histograms belowhist(d[,"Sepal.Width"], breaks = br) # illustrate scaling for specific columnhist(ds[,"Sepal.Width"], breaks = br, add=TRUE, col="red") # add histogram to current plotlegend("topright", c("orig","scaled"), fill=c("white", "red"))
Heatmaps
Heatmaps are color-coded representations of numerical matrices.
Typically the rows and columns are re-ordered according to some distance measure (default: Euclidean) and hierarchical clustering method (default: complete)
There are many tools to draw heatmaps in R. Here we use the pheatmap package to provide this powerful functionality
Code
#install.packages("pheatmap") # That's how we install new packages - more laterlibrary(pheatmap) # make packaged functions availablepaste('loaded pheatmap version:', packageVersion('pheatmap'))
[1] "loaded pheatmap version: 1.0.12"
Code
ds =scale(iris[,-5]) # scaled data for heatmapann =data.frame(Species = iris[,5]) # meta data for annotations# explicitly set rownames to retain association between data and metadatarownames(ds)=rownames(iris)rownames(ann)=rownames(iris)pheatmap(ds, annotation_row = ann,show_rownames =FALSE, )
There is many more parameters for more control - if you have lots of time read “?pheatmap”
Sending plots to files
In Rstudio, we can export figures from the “Plots” tab. On the console we can define a pdf file as a new device for all subsequent figures. This is usually done only after the image is sufficiently optimized
Code
pdf("output/heatmap.pdf") # similar for jpeg, png, ...pheatmap(ds, annotation_row = ann, show_rownames =FALSE)dev.off() # close device = pdf file
pdf
3
Goal 2: Show me all the data (in lower dimensions)
Code
M=as.matrix(iris[,-5]) # numerical data, some operations below require matrices not data frames (%*%)s=iris[,5] # species attributes (factor)
PCA Goals
simplify description of data matrix \(M\): data reduction & extract most important information
maximal variance: look for direction in which data shows maximal variation
minimal error: allow accurate reconstruction of original data
from amoeba @ https://stats.stackexchange.com/questions/2691/making-sense-of-principal-component-analysis-eigenvectors-eigenvalues
PCA with R
Code
pca =prcomp(M, scale=TRUE)
Task: What kind of object is pca?
Covariance Structure
Code
S=pca$x # score matrix = rotated and scaled data matrixpairs(S, col=s)
Code
pheatmap(cov(M)) # original covariance matrix
Code
# covariance matrix of scores is diagonal (by design)# --> principal components are uncorrelatedpheatmap(cov(S))
Notice that the higher components do not add much to the variance, so we may as well represent the transformed data in only the first two dimensions:
Code
plot(S[,1:2],pch=21, bg=s) # score-plot
Review
use heatmaps to visualize large matrices
data transformation: scale()
installing packages: pheatmap
exporting figures as publication-ready files: pdf()
dimensional reduction (PCA): prcomp()
Source Code
---title: "06: Data Visualization"author: "Thomas Manke"categories: - pheatmap - PCA---```{r, child="_setup.qmd"}```# Goal 1: Show me all the data## Scaling It's good practice to normalize the different variables```{r scaling}d=iris[,-5] # numerical iris data (without speciee) ds=scale(d) # scaled iris data (column-wise)br=seq(-5,7,by=0.5) # set common break points for the histograms belowhist(d[,"Sepal.Width"], breaks = br) # illustrate scaling for specific columnhist(ds[,"Sepal.Width"], breaks = br, add=TRUE, col="red") # add histogram to current plotlegend("topright", c("orig","scaled"), fill=c("white", "red"))```## HeatmapsHeatmaps are color-coded representations of numerical matrices. Typically the rows and columns are re-ordered according to some distance measure (default: Euclidean) and hierarchical clustering method (default: complete) There are many tools to draw heatmaps in R.Here we use the `pheatmap` package to provide this powerful functionality```{r pheatmap}#install.packages("pheatmap") # That's how we install new packages - more laterlibrary(pheatmap) # make packaged functions availablepaste('loaded pheatmap version:', packageVersion('pheatmap'))ds = scale(iris[,-5]) # scaled data for heatmapann = data.frame(Species = iris[,5]) # meta data for annotations# explicitly set rownames to retain association between data and metadatarownames(ds)=rownames(iris)rownames(ann)=rownames(iris)pheatmap(ds, annotation_row = ann, show_rownames = FALSE, )```There is many more parameters for more control - if you have lots of time read "?pheatmap" ***## Sending plots to filesIn Rstudio, we can export figures from the "Plots" tab. On the console we can define a pdf file as a new device for all subsequent figures. This is usually done only *after* the image is sufficiently optimized```{r pdf}pdf("output/heatmap.pdf") # similar for jpeg, png, ...pheatmap(ds, annotation_row = ann, show_rownames = FALSE)dev.off() # close device = pdf file```***# Goal 2: Show me all the data (in lower dimensions)```{r df2mat}M=as.matrix(iris[,-5]) # numerical data, some operations below require matrices not data frames (%*%)s=iris[,5] # species attributes (factor)```## PCA Goals* simplify description of data matrix $M$: data reduction & extract most important information* maximal variance: look for direction in which data shows maximal variation* minimal error: allow accurate reconstruction of original data 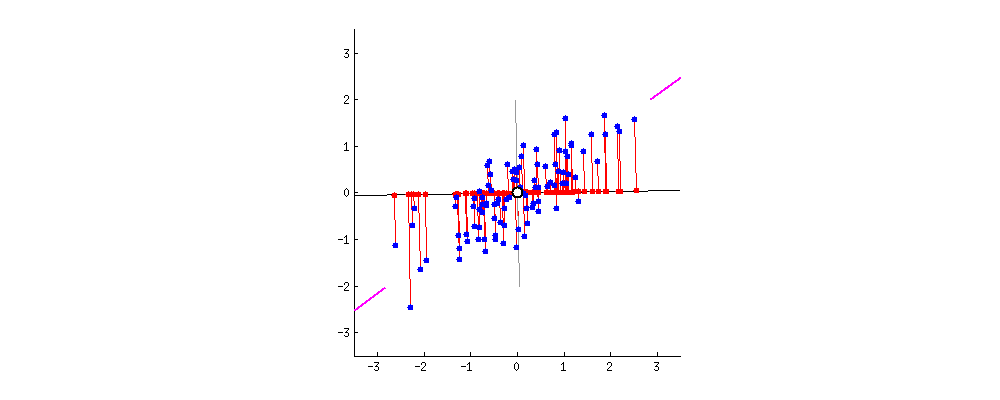from amoeba @ https://stats.stackexchange.com/questions/2691/making-sense-of-principal-component-analysis-eigenvectors-eigenvalues## PCA with R```{r run_pca}pca = prcomp(M, scale=TRUE)```**Task**: What kind of object is pca?```{r pca_obj, echo=FALSE, eval=FALSE}class(pca)typeof(pca)str(pca)methods(class="prcomp")```## Covariance Structure```{r pca_cov}S=pca$x # score matrix = rotated and scaled data matrixpairs(S, col=s)pheatmap(cov(M)) # original covariance matrix# covariance matrix of scores is diagonal (by design)# --> principal components are uncorrelatedpheatmap(cov(S)) ```Notice that the higher components do not add much to the variance, so we may as well represent the transformed data in only the **first two dimensions**:```{r plot_PC1_PC2}plot(S[,1:2],pch=21, bg=s) # score-plot```***# Review* use heatmaps to visualize large matrices* data transformation: scale()* installing packages: pheatmap* exporting figures as publication-ready files: pdf()* dimensional reduction (PCA): prcomp()